Near-Exact Recovery for Tomographic Inverse Problems via Deep Learning
von Martin Genzel, Ingo Gühring, Jan Macdonald, Maximilian März
Jahr:
2022
Publikation:
PMLR 162:7368-7381
Abstrakt:
This work is concerned with the following fundamental question in scientific machine learning: Can deep-learning-based methods solve noise-free inverse problems to near-perfect accuracy? Positive evidence is provided for the first time, focusing on a prototypical computed tomography (CT) setup.
Link:
Read the paperAdditional Information
Brief introduction of the dida co-author(s) and relevance for dida's ML developments.
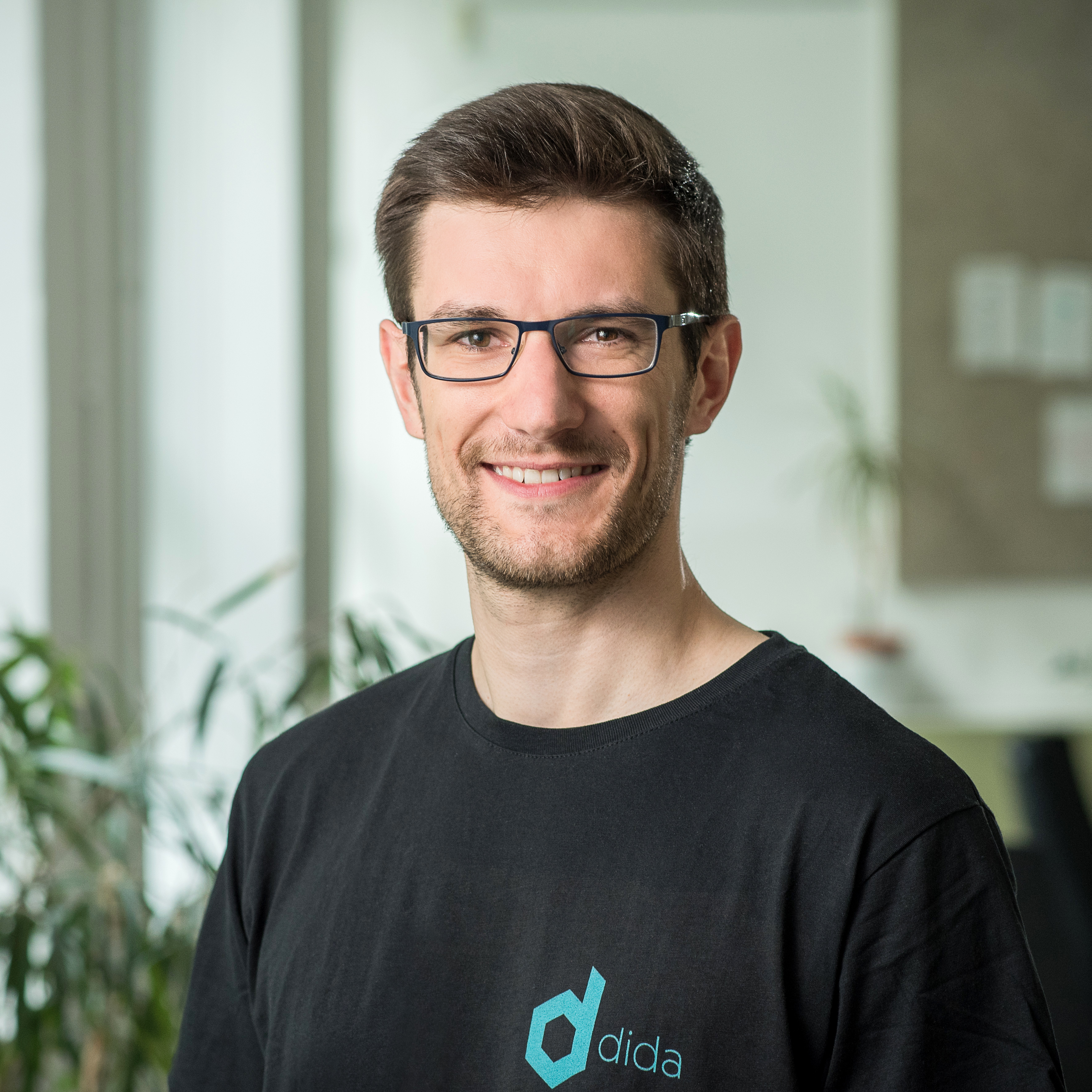
About the Co-Author
During his studies in mathematics (TU Berlin) Jan focussed on applied topics in optimization, functional analysis, and image processing. His doctoral studies (TU Berlin) explored the interplay between theoretical and empirical research on neural networks. This resulted in his PhD thesis investigating the reliability of deep learning for imaging and computer vision tasks in terms of interpretability, robustness, and accuracy. At dida he works as a Machine Learning Researcher at the interface of scientific research and software development.