dida contributed papers to NeurIPS 2022
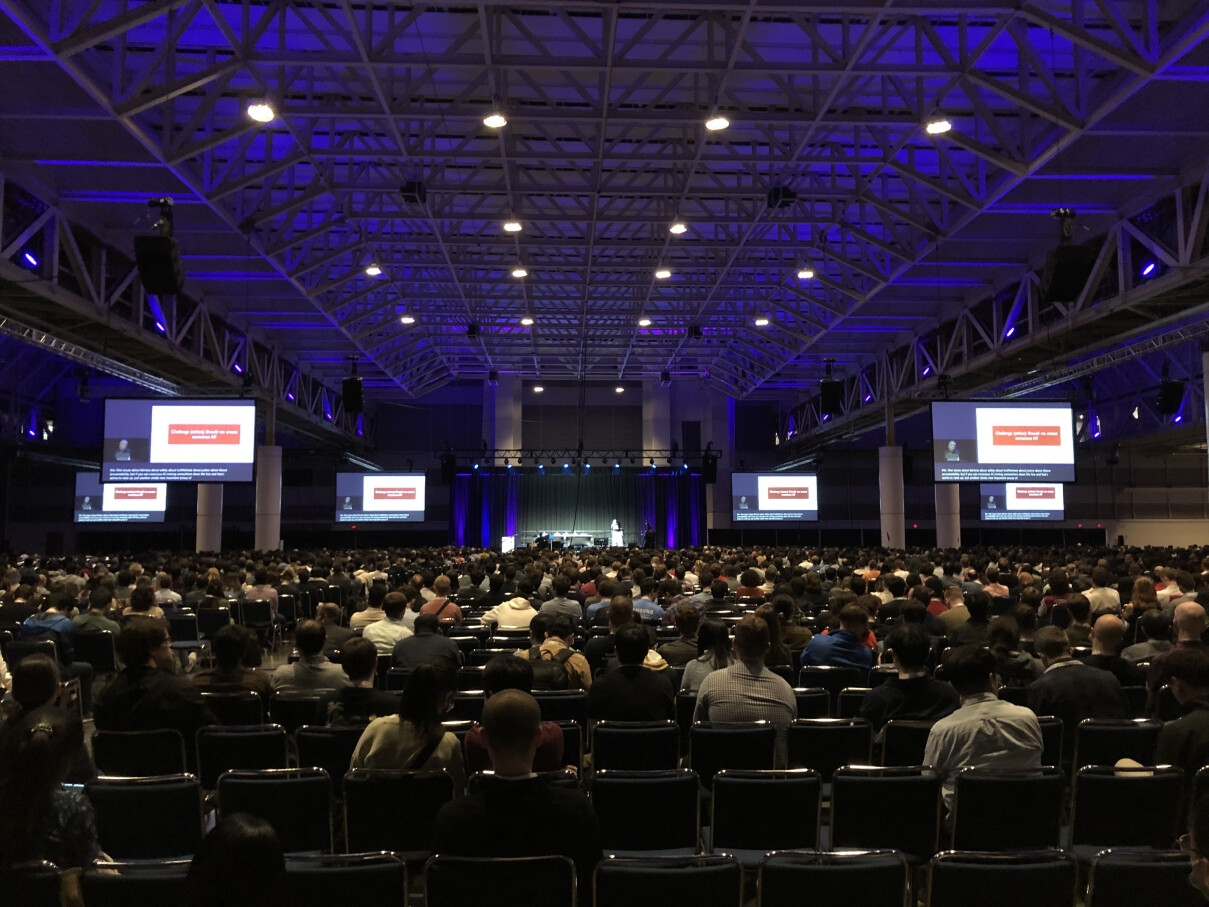
We are happy to announce that dida has made scientific contributions to this year's Conference on Neural Information Processing Systems (NeurIPS) in New Orleans. NeurIPS is one of the largest and most important conferences on innovations in machine learning and related topics.
After a contribution in terms of an accepted paper in 2020 (as well as ICML papers in 2021 and 2022), dida employees contributed a paper and workshop presentation (“An optimal control perspective on diffusion-based generative modeling”) on diffusion based generative models as well as a paper with oral presentation (“Optimal rates for regularized conditional mean embedding learning”) on nonparametric statistics to NeurIPS this year. We are proud to say that dida is able to maintain a position amongst internationally renowned researchers from leading universities as well tech companies. Out of 9634 submissions 2672 (27.7%) papers got accepted and only 184 (1.9%) received an oral contribution.
The paper “An optimal control perspective on diffusion-based generative modeling” establishes a connection between stochastic optimal control and diffusion based generative models. This perspective allows to transfer methods from optimal control theory to generative modeling and e.g. allows to derive evidence lower bound as a direct consequence of the well-known verification theorem from control theory. Further, a novel diffusion-based method for sampling from unnormalized densities is developed - a problem frequently occurring in statistics and computational sciences.
The paper “Optimal rates for regularized conditional mean embedding learning” solves two open problems related to a commonly used nonparametric regression model: we prove convergence rates for a setting when the model is misspecified (meaning that the ground truth can not be analytically represented by the model) as well as lower bounds for convergence rates of all learning algorithms solving the general underlying problem. In total, these results give conditions under which the convergence speed of the investigated model can not be beaten by any other model solving the problem.